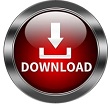
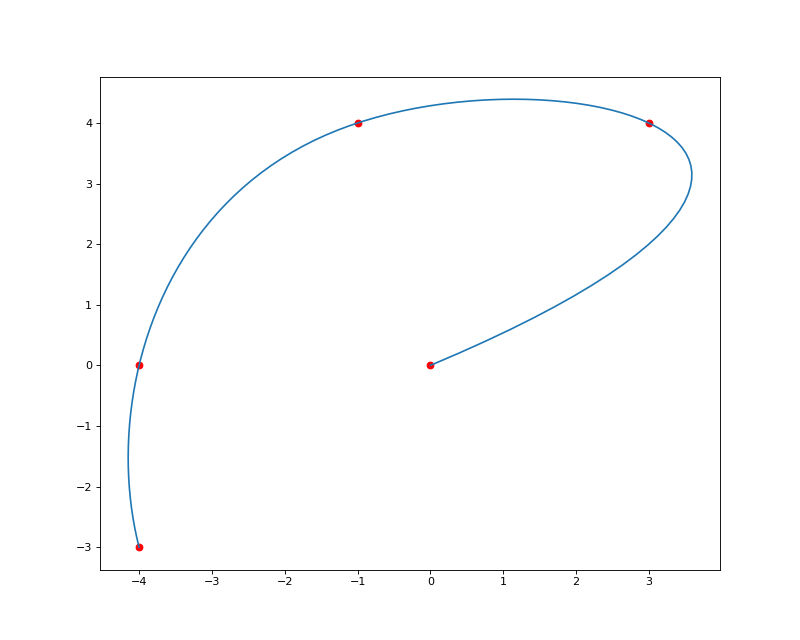
An advantage of this method is that no structural assumptions are made about the form of the plot, and the underlying distributions of the outcomes for the 2 groups do not need to be specified. The empirical method for creating an ROC plot involves plotting pairs of sensitivity versus (1−specificity) at all possible values for the decision threshold when sensitivity and specificity are calculated nonparametrically. This inherent tradeoff between sensitivity and specificity also can be demonstrated by varying the choice of threshold. However, because the optimal value of t may not be relevant to a particular application, it can be helpful to plot sensitivity and specificity over a range of values of interest, as is done with an ROC curve. One may wish to report the sum of sensitivity and specificity at the optimal threshold (discussed later in greater detail). When evaluating a continuous-scale diagnostic test, we need to account for the changes of specificity and sensitivity when the test threshold t varies. In practice, a log transformation is often applied to positive-valued marker data to obtain symmetric density functions like those depicted above.
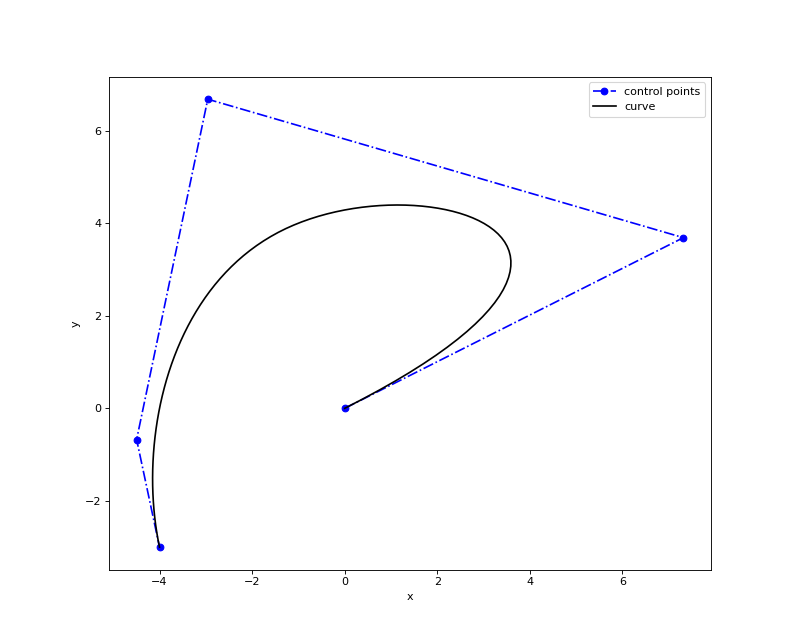
When the test is dichotomized (eg, positive if test value is greater than the threshold), both the sensitivity and specificity vary accordingly, with lower sensitivity and higher specificity as the threshold increases. For example, when the threshold value t=1, (sensitivity, specificity)=(0.72, 0.84).

Sensitivity is represented as the shaded area under the diseased distribution (B) below the same threshold of 1. The specificity of the diagnostic test is represented as the shaded area under the nondiseased distribution (A) above the arbitrary threshold t=1. The density of the diagnostic test is plotted for each of 2 populations, nondiseased (Non-D) and diseased (D), assumed to follow the binormal model with a mixture of N(0,1) and N(1.87,1.5 2), respectively. Probability density functions of a hypothetical diagnostic test that gives values on the real line. We also illustrate how these measures can be applied using the evaluation of a hypothetical new diagnostic test as an example.įigure 1. In this article, we begin by reviewing the measures of accuracy-sensitivity, specificity, and area under the curve (AUC)-that use the ROC curve. ROC analysis is a useful tool in both of these situations. Predictive modeling to estimate expected outcomes such as mortality or adverse cardiac events based on patient risk characteristics also is common in cardiovascular research. In Circulation from January 1, 1995, through December 5, 2005, 309 articles were published with the key phrase “receiver operating characteristic.” In cardiology, diagnostic testing plays a fundamental role in clinical practice (eg, serum markers of myocardial necrosis, cardiac imaging tests). Its function as a simple graphical tool for displaying the accuracy of a medical diagnostic test is one of the most well-known applications of ROC curve analysis. ROC analysis is a useful tool for evaluating the performance of diagnostic tests and more generally for evaluating the accuracy of a statistical model (eg, logistic regression, linear discriminant analysis) that classifies subjects into 1 of 2 categories, diseased or nondiseased. 1 Recently, the methodology has been adapted to several clinical areas heavily dependent on screening and diagnostic tests, 2–4 in particular, laboratory testing, 5 epidemiology, 6 radiology, 7–9 and bioinformatics. Receiver-operating characteristic (ROC) analysis was originally developed during World War II to analyze classification accuracy in differentiating signal from noise in radar detection. Customer Service and Ordering Information.Stroke: Vascular and Interventional Neurology.Journal of the American Heart Association (JAHA).Circ: Cardiovascular Quality & Outcomes.Arteriosclerosis, Thrombosis, and Vascular Biology (ATVB).
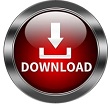